Question Machines
Question Answering systems are changing the way we find information and understand our world.
Embedded in services like Alexa, ChatGPT and Siri, they promise an answer to every question.
But how do they generate the answers to our questions?
And how much can we trust them?
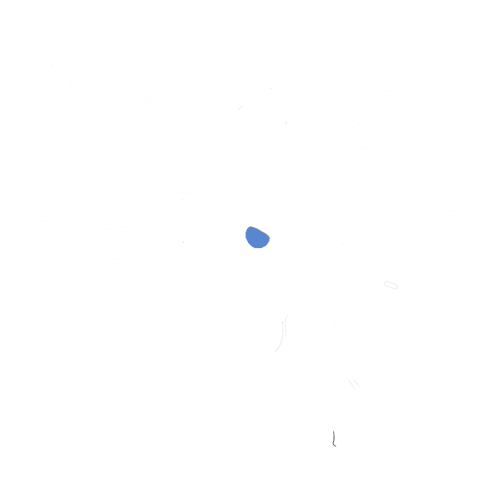
What are Question Answering Systems?
Question answering (QA) systems automate answers to questions people ask in everyday language
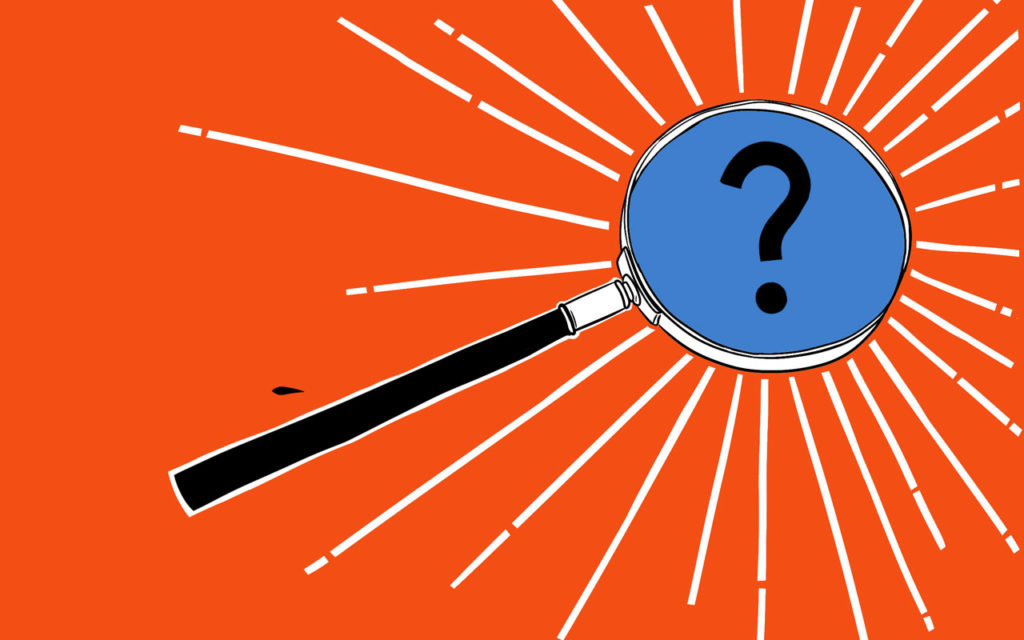
Search Engines
Search engines such as Google and Bing increasingly make use of QA systems when they present results to users.
Instead of pages of search results, search engines often present users with ‘Knowledge Panels’.
Knowledge panels draw on QA systems to provide definitive answers to questions asked by users.
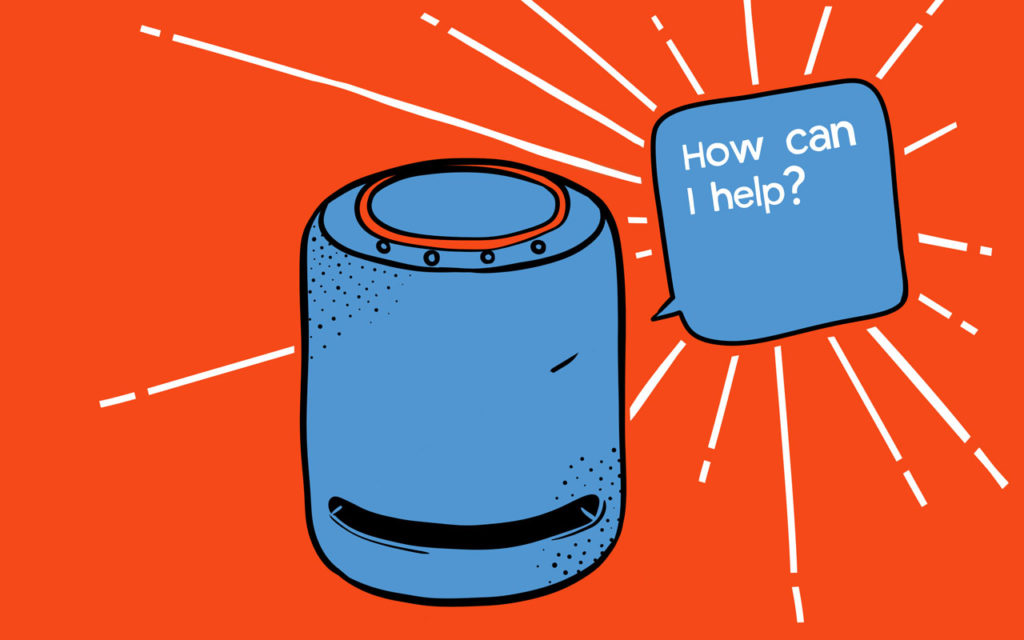
Chatbots
Chatbots and digital assistants, such as Alexa, Siri, ChatGPT and Google’s Gemini, all make use of QA systems to provide answers to questions posed by users.
These assistants are embedded into products like smart speakers, mobile phones and smart watches or layered into your online experiences through browsers or social media.
Instead of typing in a search query, users can ask questions by talking directly to the assistant.
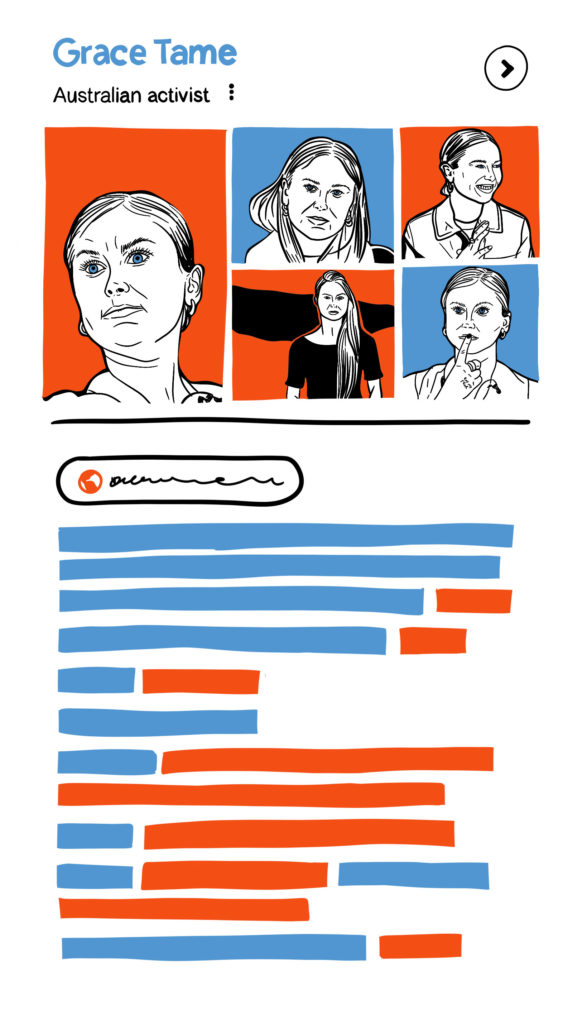
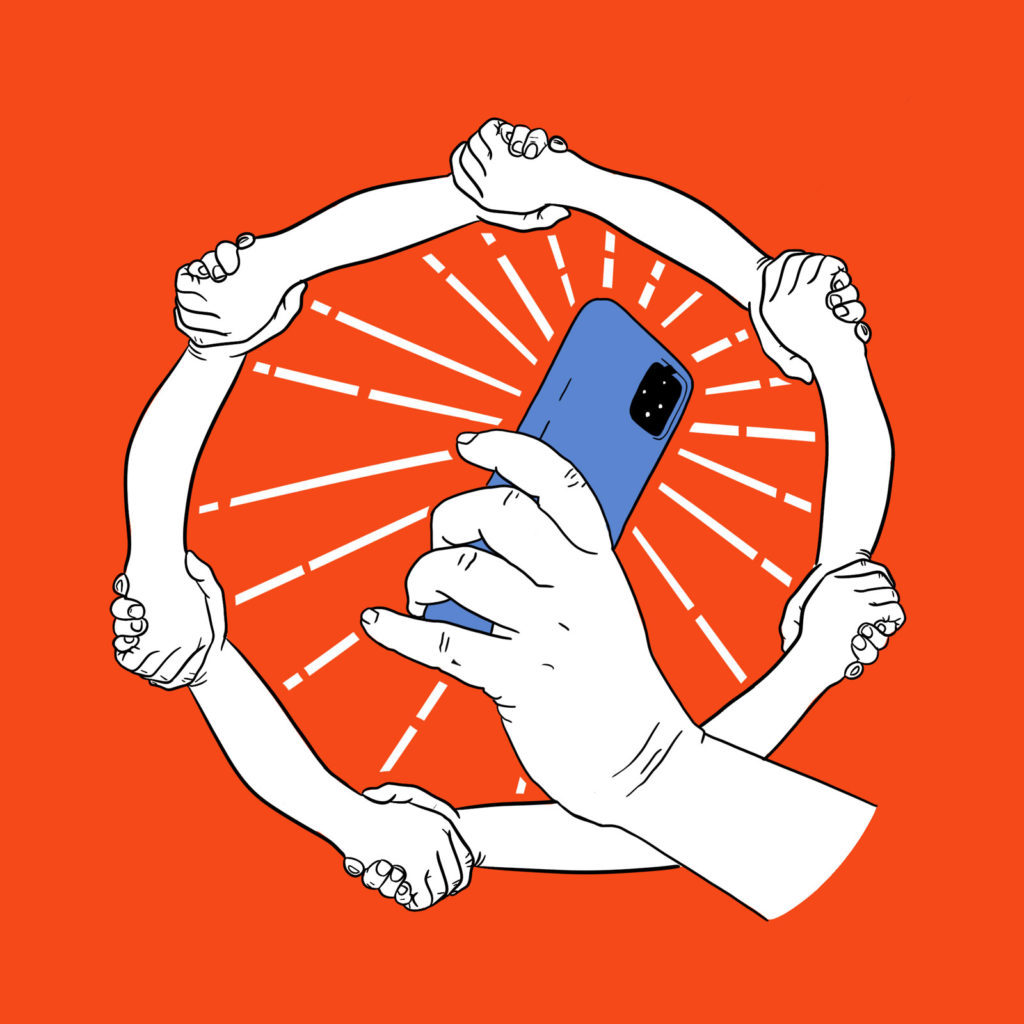
What do QA systems promise?

From maths to pop culture, Siri knows a little bit about everything
Apple

Google is leading us into an era of omnipresent, artificially intelligent assistants
Futurism

Alexa is Amazon’s all-knowing interactive voice assistant
Digital Trends
A lot of us are buying these promises. In Australia, for example...
Our research examines the implications of question answering systems, knowledge graphs and other automated knowledge systems for society
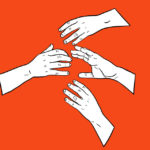
Bias
What information do these systems base their answers on?
Does this introduce new forms of bias in how the world is represented and the agency of people to determine how they are represented?
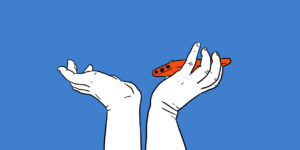
Uncertainty
All automated information is subject to varying levels of certainty.
How do automated knowledge systems reflect this in the answers they give?
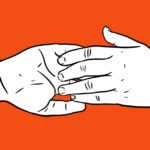
Trust
How do people evaluate the answers offered by automated knowledge systems?
What factors influence whether answers are trusted?
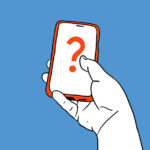
Uses
What are people using automated knowledge systems for?
Are they replacing traditional sources of information?
And what are the consequences for society?
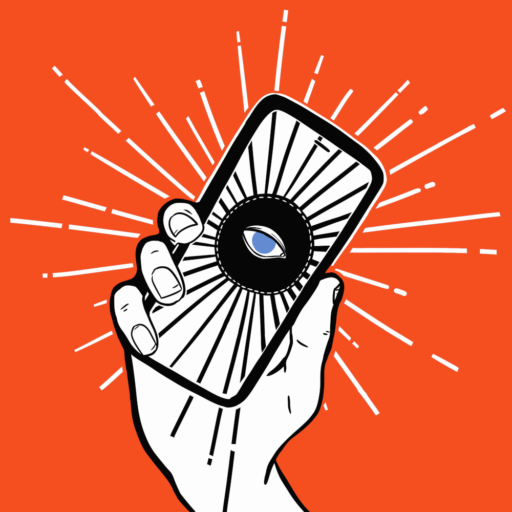